Miniaturisation and integration into existing electronics are key to the large-scale application of memristors, programmable resistors that combine memory and processor in computers. Researchers in Groningen and the US are now developing methods to get more out of their memristors.
All normal computers use a von Neumann architecture. John von Neumann, a Hungarian-American mathematician, proposed this architecture in 1945 and we still use it today. In a von Neumann architecture, memory and processor are physically separated. The computer reads data and program instructions from memory, sends them to the processor, the processor performs a calculation and sends the answer back to memory. This constant data transfer takes time and energy. No matter how fast the processor is, it has to wait for data and instructions from memory. This is called the von Neumann bottleneck. In modern applications, most of the energy is consumed by the data transfer rather than the computation itself.
New applications such as machine learning and artificial intelligence require more efficient computers. One possible solution is to integrate computing and memory. Computer scientists and materials scientists are therefore working on hardware that combines memory and computing power: so-called memristors. This is a contraction of the words memory and resistor. In short, they are programmable resistors and they have their own memory.
Matrix multiplication
’You use the laws of nature directly to perform calculations’, says Wilfred van der Wiel, professor of nanoelectronics at the University of Twente. He is co-director of BRAINS (Center for Brain-Inspired Nano Systems) and researches computation with materials. You put programmable resistors in a matrix, apply a voltage to them and measure the current that comes out. Since the current through a memristor is equal to the voltage times its conductance [I=U*G, Ohm’s law, ed], you can multiply like this. If you put several memristors in a matrix, you can add the currents together [Kirchhoff’s law of electricity, ed], so you can add them up. So you are effectively doing vector-matrix multiplication, a mathematical operation common in machine learning algorithms and neural network computation.
But how do you make an effective memristor? ’They need to be highly programmable and able to take on as many different distinguishable conductances as possible. The electronics also need to be robust and scalable. Success will depend on how you can integrate and miniaturise them’, says Van der Wiel.
’With miniaturisation, you can actually program more different values. That’s an advantage.’
Wilfred van der Wiel
Unprecedented
Researchers at the University of Groningen (RUG) published their memristors based on niobium-doped strontium titanate (Nb:SrTiO3) in Advanced Electronic Materials last February. This material system has unique properties and exhibits memristor behaviour when combined with a cobalt contact. Strontium titanate is an insulator and with doped niobium it becomes a semiconductor. The researchers used electron microscopy to show why their system can switch between higher and lower resistances and how different levels of conductivity can be programmed. They are also using a model to explain what happens when you miniaturise,” says Van der Wiel. With a smaller device, the edge effects become more important, so they actually get a larger dynamic range. So with miniaturisation you can actually program more different values. That is an advantage.
A group of American researchers recently published their memristors in Nature, in which they can program up to 2048 different values. Van der Wiel is impressed. ’This is an unprecedented number that I have never seen before.’ Moreover, these devices are fabricated in a standard 180 nm CMOS process, so they can be integrated into conventional digital electronics. ’They show, both theoretically and experimentally, that removing incomplete conduction channels dramatically reduces telegraph noise [random telegraph noise, RTN, ed.], making it possible to program so many distinguishable conduction levels. Their noise reduction method can also be applied to other memristor materials.’
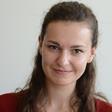
Nog geen opmerkingen